Traj-MAE: Masked Autoencoders for Trajectory Prediction
International Conference on Computer Vision(ICCV) 2023
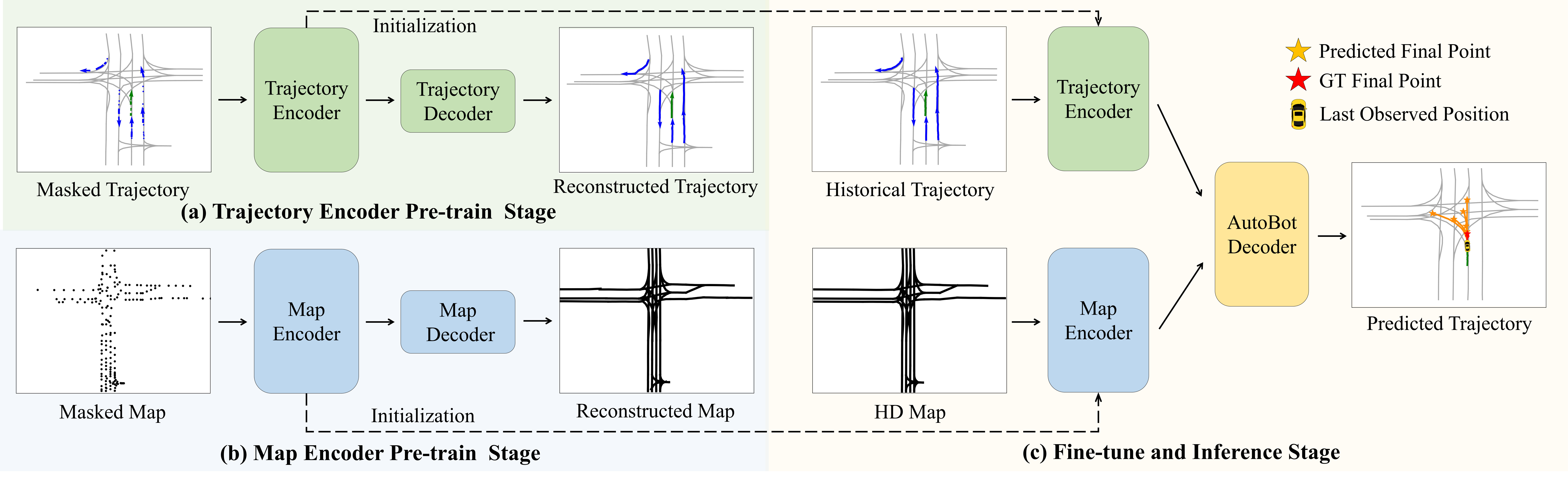
Overview
Trajectory prediction has been a crucial task in building a reliable autonomous driving system by anticipating possible dangers. One key issue is to generate consistent trajectory predictions without colliding. To overcome the challenge, we propose an efficient masked autoencoder for trajectory prediction (Traj-MAE) that better represents the complicated behaviors of agents in the driving environment. Specifically, our Traj-MAE employs diverse masking strategies to pre-train the trajectory encoder and map encoder, allowing for the capture of social and temporal information among agents while leveraging the effect of environment from multiple granularities. To address the catastrophic forgetting problem that arises when pre-training the network with multiple masking strategies, we introduce a continual pre-training framework, which can help Traj-MAE learn valuable and diverse information from various strategies efficiently. Our experimental results in both multi-agent and single-agent settings demonstrate that Traj-MAE achieves competitive results with state-of-the-art methods and significantly outperforms our baseline model.
Citation
@inproceedings{chen2023traj, title={Traj-MAE: Masked Autoencoders for Trajectory Prediction}, author={Chen, Hao and Wang, Jiaze and Shao, Kun and Liu, Furui and Hao, Jianye and Guan, Chenyong and Chen, Guangyong and Heng, Pheng-Ann}, booktitle = {2023 International Conference on Computer Vision (ICCV)}, year={2023} }